Elon Musk and Mark Zuckerberg recently enjoyed a high profile spat about AI’s potential for apocalyptic side-effects. In a rather infantile exchange conducted via the gossiping journalists of the tech playground, Mark said that he thought Elon was a crybaby for whining about superintelligence, whilst Elon accused Mark of being a dummy who didn’t know what he was talking about.
Their perspectives are both supported by a variety of weighty brains. In Musk’s corner, warning of the potential for misalignment between man and machine are Stuart Russell and Nick Bostrom; in Zuckerberg’s, Andrew Ng and Yann LecCun express deep skepticism about the imminent arrival of a humanity-threatening agent. One of the kernels of the debate is a simple question: how clever are our machines going to get in the next couple of decades?*
This debate has been spurred by a recent spate of successes. In particular, algorithms have started to beat humans at games that had previously been considered too complex for computers, such as Go (cracked by Google DeepMind’s AlphaGo, which recently attained a ‘divine’ ranking from the South Korean Go association), and poker. As these milestones fall by the wayside, it’s natural to ask at what point we will start to see machines that supercede humans in so many ways that we need to start worrying about potential side-effects.
Predicting the course of technological advance is always fraught. As little as 6 years before the invention of the atom bomb, luminaries of physical science like Niels Bohr were declaring it impossible. But perhaps in assessing the intelligence of machines we are confronting another obstacle: our own concept of intelligence.
The study of human intelligence has a chequered past. Much of the early work set out to map differences in intelligence according to a eugenic agenda: to prove that some races were systematically cleverer than others. From this twisted beginning arose a surprising conclusion. Over and over again, people have found that you can summarise people’s intelligence with more or less a single number. Today, we know this number as IQ.
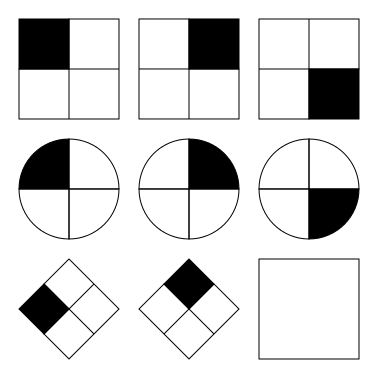
How do you measure intelligence? Intuitively, it seems like there might be lots of different kinds of intelligence, and so we should have different measures of each. Maybe one test for language; another for remembering things; another for mathematics and so on. One of the first people to systematically map out the results of these different kinds of test was Charles Spearman (he of the correlation coeffficient). His surprising conclusion was that a given person did the same in more or less all of these tasks. If you knew how good somebody was at maths, you could make a pretty accurate guess at how many words they knew; if you had a measure of how well they remembered a list of numbers, you could estimate how good they were at imagining what a shape looked like when it was rotated.
‘ All examination, therefore, in the different sensory, school, or other specific intellectual faculties, may be regarded as so many independently obtained estimates of the one great common Intellective Function’
Over an illustrious career Spearman confirmed that a single factor, which came to be known as ‘Spearman’s g’, largely explained human intelligence. Technically speaking, he showed that a single component captures most of the variance in intelligence, measured across a wide range of tasks. Furthermore, we now know that if you measure somebody’s ‘g’, you can make reasonable predictions about real-world measures of success, such as educational attainment and employment prospects.
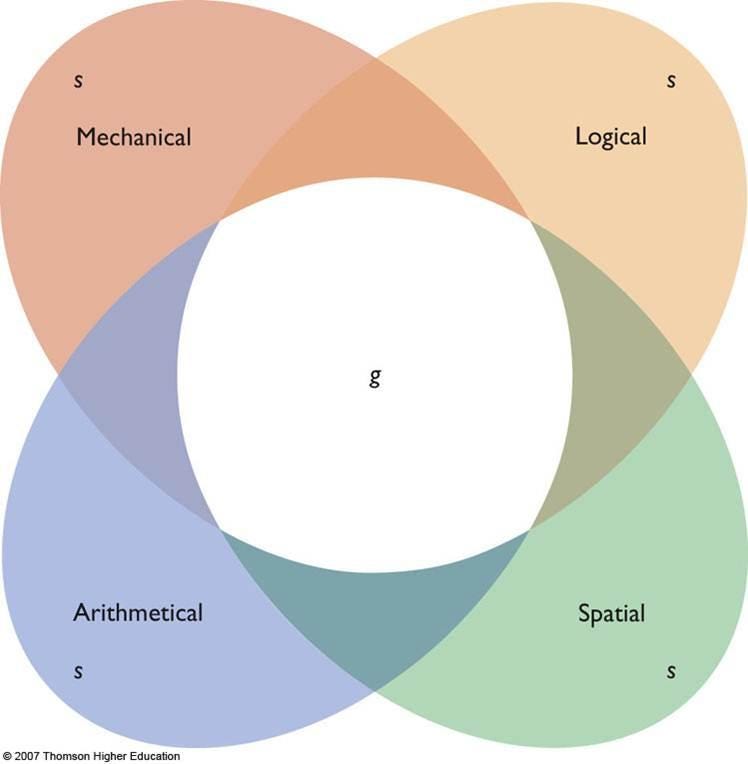
We don’t know why this is. We do know, however, that our brains are very good at learning about patterns like this one. The brain is a supreme pattern recogniser; this is why people see the Virgin Mary on pieces of burnt toast, or hear messages when songs are played backwards.
We are assessing the intelligence of other people every day of our lives. When the time comes to assess the intelligence of machines, we fall back upon the mental models we use to assess humans. This isn’t surprising: in fact it’s an example of generalisation, using data acquired in one situation to understand a different one.
[Ironically, this is something that current computers are very bad at. A machine that has learned how to play the Atari game pong fails spectacularly if the paddle is moved up a few pixels, whilst humans can switch between tennis, baseball, and badminton with ease.]
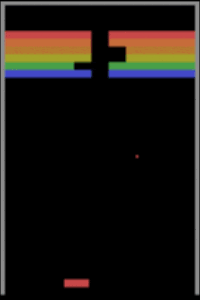
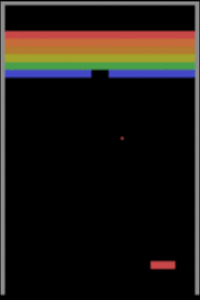
So when we are asked to assess whether a machine like AlphaGo is a portent of superintelligence, perhaps we fall back upon a our understanding of the statistics of intelligence. For humans, being good at one type of task means you’re probably pretty good at others. So seeing an algorithm conquer a human in one of the most intellectually demanding games humanity has devised is scary. And perhaps that’s because if a human is very good at Go, it probably means she’s good at lots of other things. In fact, she’s probably got a very high IQ.
To emphasise this point further, consider AlphaGo’s grandfather, child prodigy and DeepMind founder Demis Hassabis. Demis is a chess grandmaster. But, and you already know this bit, he’s not just good at chess: he’s designed fantastically successful computer games, he’s a world champion at strategy games, and he’s built the worlds best AI lab whilst working as a neuroscientist. He’s an unusually talented guy, just as AlphaGo is an unusually talented algorithm. But Demis is talented in lots of ways, just as the study of human intelligence suggests he should be: AlphaGo is not.
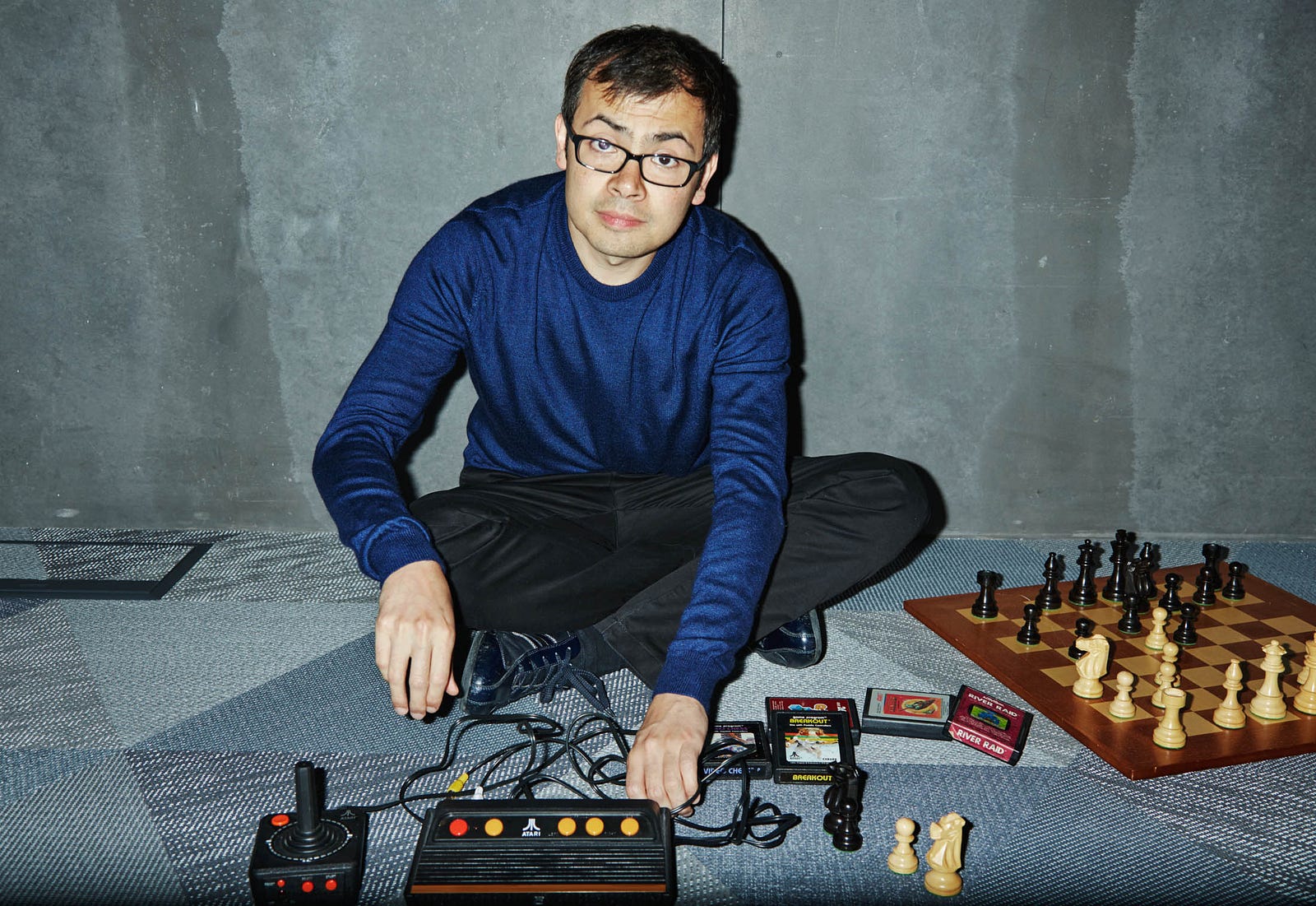
AlphaGo is designed by humans, rather than evolution. And it is designed to do one thing, which it does extraordinarily well. This is, broadly speaking, true of all of the algorithms we have today. They are trained for long periods of time, consume huge amounts of data, and learn to perform a single task.
So: perhaps it’s too early to start worrying about machine intelligence. Perhaps we’ve been fooled by our own intuitions about intelligence, which work so well when assessing other humans, but might be misleading when applied to computer programs. In the meantime, as pointed out by leading members of the community like Francois Chollet, we have plenty of more tangible problems to confront with AI: like ensuring transparency and oversight, preventing biases, and dealing with the societal effects of rising robot employment.
One way to better assess our progress, moving beyond intuition and emotion, might be to establish agreed-upon checkpoints at which we should take stock of the state of machine intelligence. Clearly our own concept of IQ is a poor guide; in fact, a relatively simple computer program would ace an IQ test. Perhaps we need a new set of AIQ tests, assessing flexibility and reasoning across multiple domains, and for which training data is very sparse or absent. By breaking up the path to superintelligence into a series of well-defined waypoints, we might be able to measure our progress in a more principled way — and avoid any more fights between Elon and Mark.
*A study conducted earlier this year by the Future of Humanity Institute (and summarised by Scott Alexander at Slate Star Codex) suggests that this spectrum of opinion is representative of the community as a whole. There’s little agreement on when various AI milestones will occur.